“All models are wrong; some are useful.” – George Box
We have spent three articles (article 1, article 2, article 3) explaining how catastrophe models provide a tool for much-needed innovation to the global insurance industry. Catastrophe models have covered for the lack of experience with many losses and let insurers properly price and underwrite risks, manage portfolios, allocate capital and design risk management strategies. Yet for all the practical benefits CAT models have infused into the industry, product innovation has stalled.
The halt in progress is a function of what models are and how they work. In fairness to those who do not put as much stock in the models as a useful tool, it is important to speak of the models’ limitations and where the next wave of innovation needs to come from.
Model Design
Models are sets of simplistic instructions that are used to explain phenomena and provide relevant insight on future events (for CAT models – estimating future catastrophic losses). We humans start using models at very early ages. No one would confuse a model airplane with a real one; however, if a parent wanted to simplify the laws of physics to explain to a child how planes fly, then a model airplane is a better tool than, say, a physics book or computer-aided design software. Conversely, if you are a college student studying engineering or aerodynamics, the reverse is true. In each case, we are attempting to use a tool – models of flight, in this instance – to explain how things work and to lend insight into what could happen based on historical data so that we can merge theory and practice into something useful. It is the constant iteration between theory and practice that allows an airplane manufacturer to build a new fighter jet, for instance. No manufacturer would foolishly build an airplane based on models no matter how scientifically advanced those models are, but those models would be incredibly useful in guiding the designers to experimental prototypes. We build models, test them, update them with new knowledge, test them again and repeat the process until we achieve desired results.
The design and use of CAT models follows this exact pattern. The first CAT models estimated loss by first calculating total industry losses and then proportionally allocating losses to insurers based on assumption of market share. That evolved into calculating loss estimates for specific locations at specific addresses. As technology advanced into the 1990s, model developers harnessed that computing power and were able to develop simulation programs to analyze more data, faster. The model vendors then added more models to cover more global peril regions. Today’s CAT models can even estimate construction type, height and building age if an insurer does not readily have that information.
As catastrophic events occur, modelers routinely compare the actual event losses with the models and measure how well or how poorly the models performed. Using actual incurred loss data helps calibrate the models and also enables modelers to better understand the areas in which improvements must be made to make them more resilient.
However, for all the effort and resources put into improving the models (model vendors spend millions of dollars each year on model research, development, improvement and quality assurance), there is still much work to be done to make them even more useful to the industry. In fact, virtually every model component has its limitations. A CAT model’s hazard module is a good example.
The hazard module takes into account the frequency and severity of potential disasters. Following the calamitous 2004 and 2005 U.S. hurricane seasons, the chief model vendors felt pressure to amend their base catalogs with something to reflect the new high-risk era we were in, that is, taking into account higher-than-average sea surface temperatures. These model changes dramatically affected reinsurance purchase decisions and account pricing. And yet, little followed. What was assumed to be the new normal of risk taking actually turned into one of the quietest periods on record.
Another example was the magnitude 9.0, 2011 Great Tōhuko Earthquake in Japan. The models had no events even close to this monster earthquake in their event catalogs. Every model clearly got it wrong, and, as a result, model vendors scrambled to fix this “error” in the model. Have the errors been corrected? Perhaps in these circumstances, but what other significant model errors exist that have yet to be corrected?
CAT model peer reviewers have also taken issue with actual event catalogs used in the modeling process to quantify catastrophic loss. For example, a problem for insurers is answering the type of question of: What is the probability of a Category 5 hurricane making landfall in New York City? Of course, no one can provide an answer with certainty. However, while no one can doubt the significance of the level of damage an event of that intensity would bring to New York City (Super Storm Sandy was not even a hurricane at landfall in 2012 and yet caused tens of billions of dollars in insured damages), the critical question for insurers is: Is this event rare enough that it can be ignored, or do we need to prepare for an event of that magnitude?
To place this into context, the Category 3, 1938 Long Island Express event would probably cause more than $50 billion in insured losses today, and that event did not even strike New York City. If a Category 5 hurricane hitting New York City was estimated to cause $100 billion in insured losses, then knowing whether this was a 1-in-10,000-year possibility or a 1-in-100-year possibility could mean the difference between solvency and insolvency for many carriers. If that type of storm was closer to a 1-in-100-year probability, then insurers have the obligation to manage their operations around this possibility; the consequences are too grave, otherwise.
Taking into account the various chances of a Category 5 directly striking New York City, what does that all mean? It means that adjustments in underwriting, pricing, accumulated capacity in that region and, of course, reinsurance design all need to be considered -- or reconsidered, depending on an insurer’s present position relative to its risk appetite. Knowing the true probability is not possible at this time; we need more time and research to understand that. Unfortunately for insurers, rating agencies and regulators, we live in the present, and sole reliance on the models to provide “answers” is not enough.
Compounding this problem is that, regardless of the peril, errors exist in every model’s event catalog. These errors cannot even be avoided, and the problem escalates where our paucity of historical recordings and scientific experiments limit our industry’s ability to inch us closer and closer to greater certainty.
Earthquake models still lie beyond a comfortable reach of predictability. Some of the largest and most consequential earthquakes in U.S. history have occurred near New Madrid, MO. Scientists are still wrestling with the mechanics of that fault system. Thus, managing a portfolio of properties solely dependent on CAT model output is foolhardy at best. There is too much financial consequence from phenomena that scientists still do not understand.
Modelers also need to continuously assess property vulnerability when it comes to taking into consideration various building stock types with current building codes. Assessing this with imperfect data and across differing building codes and regulations is difficult. That is largely the reason that so-called “vulnerability curves” oftentimes are revised after spates of significant events. Understandably, each event yields additional data points for consideration, which must be taken into account in future model versions. Damage surveys following Hurricane Ike showed that the models underestimated contents vulnerability within large high-rises because of water damage caused by wind-driven rain.
As previously described, a model is a set of simplified instructions, which can be programmed to make various assumptions based on the input provided. Models, therefore, fall into the Garbage In – Garbage out complex. As insurers adapt to these new models, they often need to cajole their legacy IT systems to provide the required data to run the models. For many insurers, this is an expensive and resource-intensive process, often taking years.
Data Quality’s Importance
Currently, the quality of industry data to be used in such tools as CAT models is generally considered poor. Many insurers are inputting unchecked data into the models. For example, it is not uncommon that building construction type, occupancy, height and age, not to mention a property’s actual physical address, are unknown! For each property whose primary and secondary risk characteristics are missing, the models must make assumptions regarding those precious missing inputs – even regarding where the property is located. This increases model uncertainty, which can lead to inaccurate assessment of an insurer's risk exposure.
CAT modeling results are largely ineffective without quality data collection. For insurers, the key risk is that poor data quality could lead to a misunderstanding regarding what their exposure is to potential catastrophic events. This, in turn, will have an impact on portfolio management, possibly leading to unwanted exposure distribution and unexpected losses, which will affect both insurers’ and their reinsurers’ balance sheets. If model results are skewed as a result of poor data quality, this can lead to incorrect assumptions, inadequate capitalization and the failure to purchase sufficient reinsurance for insurers. Model results based on complete and accurate data ensures greater model output certainty and credibility.
The Future
Models are designed and built based on information from the past. Using them is like trying to drive a car by only looking in the rear view mirror; nonetheless, catastrophes, whether natural or man-made, are inevitable, and having a robust means to quantify them is critical to the global insurance marketplace and lifecycle.
Or is it?
Models, and CAT models in particular, provide a credible industry tool to simulate the future based on the past, but is it possible to simulate the future based on perceived trends and worst-case scenarios? Every CAT model has its imperfections, which must be taken into account, especially when employing modeling best practices. All key stakeholders in the global insurance market, from retail and wholesale brokers to reinsurance intermediaries, from insurers to reinsurers and to the capital markets and beyond, must understand the extent of those imperfections, how error-sensitive the models can be and how those imperfections must be accounted for to gain the most accurate insight into individual risks or entire risk portfolios. The difference in a few can mean a lot.
The next wave of innovation in property insurance will come from going back to insurance basics: managing risk for the customer. Despite model limitations, creative and innovative entrepreneurs will use models to bundle complex packages of risks that will be both profitable to the insurer and economical to the consumer. Consumers desiring to protect themselves from earthquake risks in California, hurricane risks in Florida and flood risks on the coast and inland will have more options. Insurers looking to deploy capital and find new avenues of growth will use CAT models to simulate millions of scenarios to custom create portfolios optimizing their capacity and create innovative product features to distinguish their products against competitors. Intermediaries will use the models to educate and craft effective risk management programs to maximize their clients’ profitability.
For all the benefit CAT models have provided the industry over the past 25 years, we are only driving the benefit down to the consumer in marginal ways. The successful property insurers of the future will be the ones who close the circle and use the models to create products that make the transfer of earthquake, hurricane and other catastrophic risks available and affordable.
In our next article, we will examine how we can use CAT models to solve some of the critical insurance problems we face.
Model Errors in Disaster Planning
This article is the fourth in a series on how the evolution of catastrophe models provides a foundation for innovation.
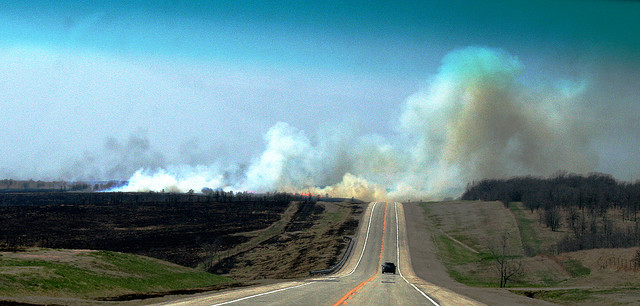