- Size and scope – Big data is getting bigger and faster. With connected cars, homes and buildings, and machines, the amount of data is increasing exponentially. Investments in IoT and Industrial IoT, 5G and other related areas will only increase the speed and amount of data. With this increased volume and velocity, we will not be able to generate meaningful insights from all of this data without advanced analytics and artificial intelligence.
- Big data technology – Big data technology is moving from Hadoop to streaming architectures to hybrid “translytical” databases. While concepts like “data lakes” and NoSQL databases mature, new technologies like Apache Spark, Tez, Storm, BigTop and REEF, among others, are creating a constant flow of new tools, which adds to a sense of “big data in flux.”
- Democratization – The democratization of data, business intelligence and data science is accelerating. Essentially, this means that anybody in a given organization with the right permissions can use any dataset, slice and dice the data, run analysis and create reports with very little help from IT or data scientists. This creates expectations for timely delivery, and business analysts can no longer hide behind IT timelines and potential delays.
- Open source movement – The open source revolution in data, code and citizen data scientist is accelerating access to data and generation of insights. Open source tools are maturing and finding their way into commercial vendor solutions, and the pace of open source tool creation is continuing unabated; the Apache Software Foundation lists more than 350 current open source initiatives. This steady stream requires data engineers and data scientists to constantly evaluate tools and discover new ways of data engineering and data science.
- Ubiquitous intelligence – Advanced analytics – especially various types of artificial intelligence areas (reference to my AI report post) – is evolving and becoming ubiquitous intelligence. AI can now interact with us through natural language, speak to us, hear us, see the world and even feel objects. As a result, it will start seamlessly weaving itself into many of our day-to-day activities, such as using a search engine or sorting our email, recommending things to buy based on our preferences and needs, seeing the world and guiding us through our interaction with other people and things without our even being aware of its doing so. This will further heighten our sense of disruption and constant change.
- Deep learning – Deep learning, a subset of the machine learning family (which itself is just one area of AI), has been improving in speed, scale, accuracy, sophistication and the scope of problems it addresses. Unlike previous techniques, which were specific to the different type of data (e.g., text, audio, image), deep learning techniques have been applied across all different types of data. This has contributed to reduced development time and greater sharing and broadened the scope of innovation and disruption.
- MLaaS – Machine learning, cloud computing and open source movement are converging to create Machine Learning as a Service (MLaaS). This not only decreases the overall variable costs of using AI but also provides large volumes of data that the machine learning systems can further exploit to improve their accuracy, resulting in a virtuous cycle.
- Funding – Big data funding peaked in 2015. However, funding for artificial intelligence, especially machine learning and deep learning, has continued to attract increasingly significant investments. In the first half of this year, more than $3.6 billion has been invested in AI and machine learning. This increased funding has attracted great talent to explore difficult areas of AI that will be disruptors of the future economy.
- Center of Excellence: As organizations continue to obtain good ROI from their initial pilots and proof-of-concepts in analytics, automation and AI efforts, they are increasingly looking toward setting up centers of excellence where they can train, nurture and grow the talent. The exact role of the center changes based on the overall organizational culture and how the rest of their business operates – centralized, federated or decentralized.
- Competitive landscape – The big data landscape continues to grow, and the AI landscape is expanding rapidly. Deep learning companies are growing the fastest across multiple sectors. Competition among startups – as well as incumbents that want to stay ahead of potential disruption – is creating a vibrant ecosystem of partnerships and mergers and acquisitions that further the disruptive cycle.
10 Trends on Big Data, Advanced Analytics
Big data is getting bigger and faster. We will not be able to generate meaningful insights without advanced analytics and artificial intelligence.
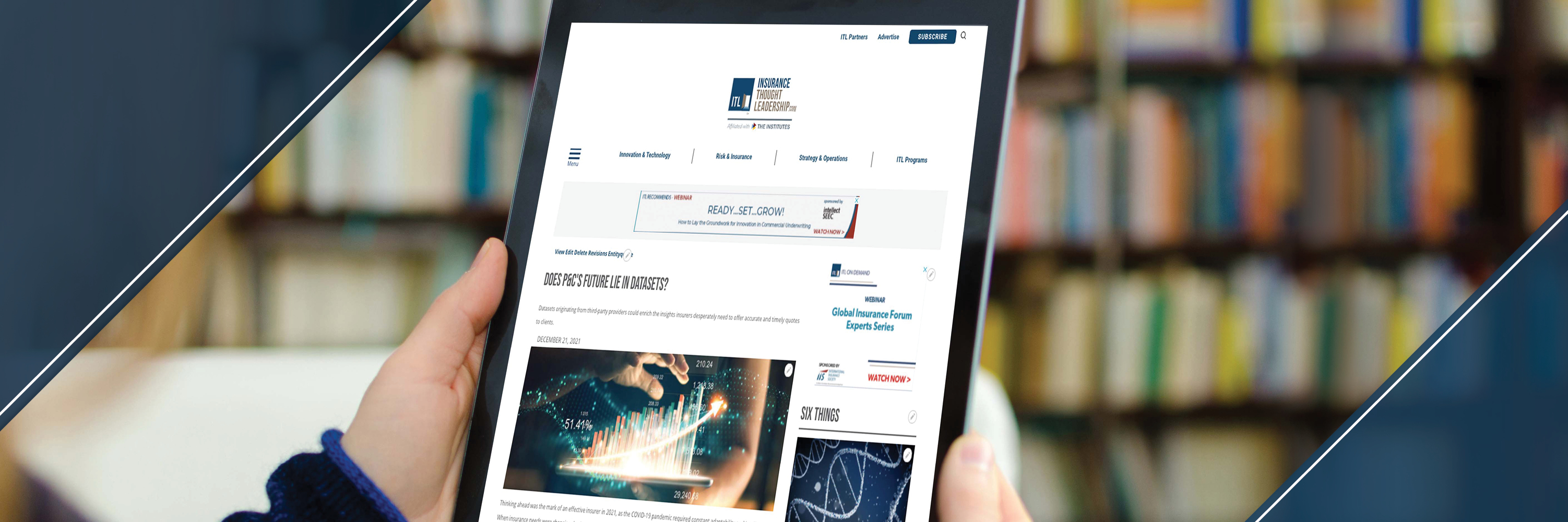