Smart technologies, artificial intelligence (AI) and machine learning are all on the agenda for insurers. But how intelligent are the systems really?
Google, Amazon or Facebook have led the way, and data is the currency of the future. This is particularly true for insurers, because, if you know your customers' behavior and life situation, you can price risks more accurately, minimize fraud and better meet customer expectations. Because processes are data-intensive and driven by repetition, many customer inquiries, damage reports or data analyses could theoretically be standardized and automated -- ideal prerequisites for using intelligent machines.
Nevertheless, the insurance industry is one of the few industries that has barely arrived in the 21st century. Paper-based processes and outdated IT systems with incompatible interfaces are the rule rather than the exception. For this reason, according to a study by the strategy consultancy Bain, companies have so far concentrated on using smart algorithms to make individual sales processes more efficient or to provide more targeted information.
According to Bain, the greatest potential slumbers in downstream areas: The property and casualty (P&C) insurers alone could increase premium income by almost 25% and reduce costs by almost 30% through consistent digitalization. The greatest savings are possible in claims settlement and acquisition costs.
See also: Strategist’s Guide to Artificial Intelligence
When IBM's Deep Blue chess computer won against the then-reigning world champion Garri Kasparov more than 20 years ago, this was celebrated as historic. However, Deep Blue did not win through cognitive intelligence, but because it could calculate all conceivable moves.
Today's AI methods, which go beyond just computing capacity and are based on algorithms that use large amounts of data to learn to carry out tasks without pre-defined rules, have so far largely failed in the insurance world. The reason: Very few insurers have an IT infrastructure that would allow customer data to be bundled over the entire contract term and all interfaces. The systems therefore do not have sufficient high-quality and correctly classified training data with which to learn the algorithms.
Instead, most companies rely on systems that obey fixed rules. For example, some providers work with chatbots to process customer inquiries faster and more automatically. Digital language assistants, which process natural language and interact directly with the customer, point in a similar direction. Whether in writing or by telephone — the basic principle is the same: Employees are relieved of routine tasks so that they have more time for those questions where personal contact is really necessary.
Nevertheless, experts agree that AI will revolutionize the insurance industry in the medium to long term. Smart algorithms will help identify insurance fraud faster and assess risks more accurately. This allows AI to be used to create personalized products. In combination with sensors and the "Internet of Things" (IoT), AI also helps to prevent fraud.
See also: And the Winner Is…Artificial Intelligence!
Together, these many possibilities will lead to insurance products that are much more individual and fair for the collective. If insurers can refine the risk profiles of their customers on the basis of the customer's history and behavior, they can determine whether this customer is trustworthy. The subjective decisions of individual experts would then be opposed by objective, trained systems that could not be impressed by emotions or stress.
Today, on the other hand, customers quickly end up in a drawer based on their profession or place of residence that does not correspond to their actual risk profile. Artificial intelligence could calculate trust on the basis of data and synthesize it, so to speak. Customers who are trustworthy can then benefit, for example, from lower prices or faster claims processing.
Untapped Potential of Artificial Intelligence
Few insurers have sufficient high-quality and correctly classified training data with which to learn AI algorithms.
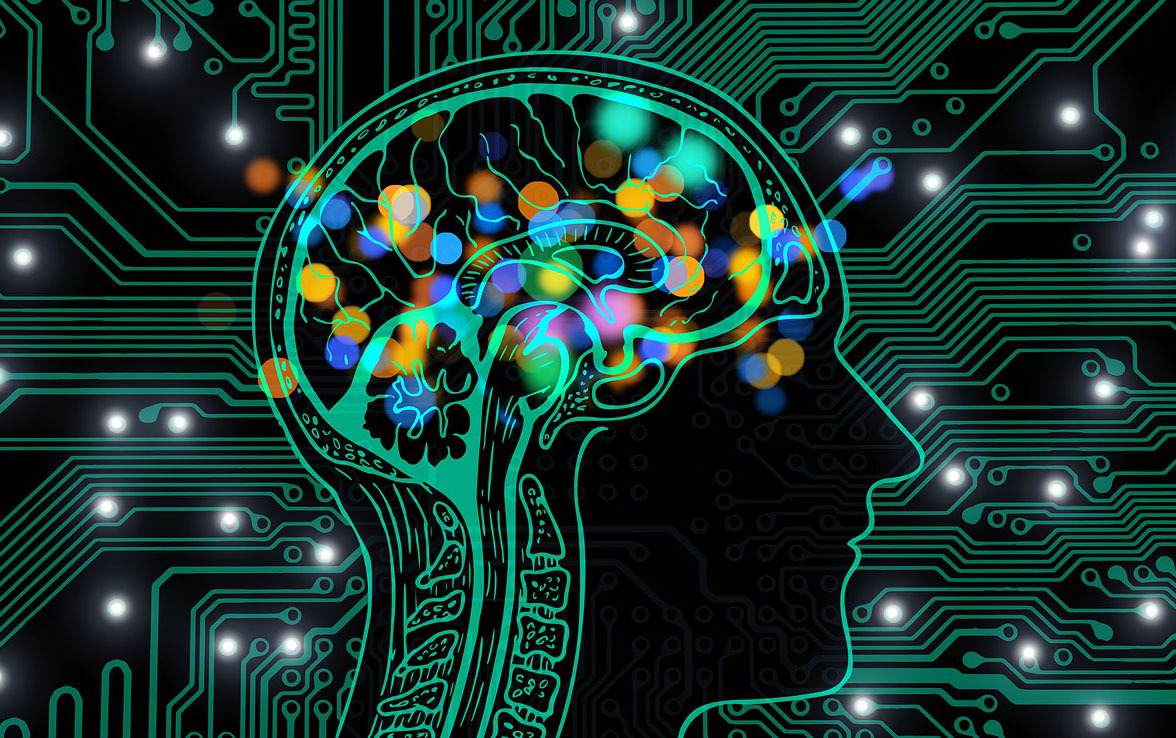