Labeled as slow movers, insurers are perhaps the only ones with live investments in generative AI (GenAI) across the board. The data at the industry’s disposal has hit critical mass, which, coupled with its proclivity to meet customers at the point of need, sets the stage to cash in efficiency gains up to 10% to 20% with GenAI.
However, as the industry pivots to an ecosystem, witnessing decentralized distribution and customer acquisition with insurtechs and embedded insurance, it will need GenAI to scale up across the value chain, not just at the enterprise level, to be transformational.
See also: Balancing AI and the Future of Insurance
Pockets of Opportunity to Plug in GenAI
We see the industry responding to a new reality with these trends, setting the stage for multiple, valuable GenAI use cases:
- Behavior-Linked Premiums: Despite collecting scads of customer data to assess risks, insurers still face the possibility of information asymmetry. Moral hazard, where the policyholder retains critical knowledge that leads to the insurer onboarding excessive risks, is a case in point. Integrating with alternate data sources (social media, telecoms, automobile manufacturers, hospitals, etc.) can offer a breakthrough. For instance, for auto insurance, large language models (LLMs) trained on alternate data sources can generate dynamic quotes based on a customer’s historical traffic tickets, auto repairs and most-frequented routes as per in-car navigation systems or ride-sharing apps. This dynamic quote turns into "pay-as-you-drive," conveyed to the customer in real-time through GenAI, paving the way for behavior-linked premiums that reward good behavior and push customers to de-risk.
- Real-Time Risk Assessment: Given the rise of the Internet of Things, insurers have newer avenues to price in risk. For instance, smartwatches and fitness trackers can help gauge a customer’s physical activity and estimate the quality of life based on recorded calorie intake. A GenAI-led self-assessment portal can query the customer on vital health information and, if required, redirect them to a local clinic for further investigation. After issuing a policy, the insurer can correlate real-time data streaming from fitness trackers to dynamically adjust premiums based on changing risk profiles. Another example would be using drones to assess a landscape for property insurance with real-time footage to assess the estate, helping insurers offer dynamic coverage based on usage, upkeep and the possibility of a calamity.
- Streamlined Distribution: With granular behavioral information and real-time premiums, insurers can embed offerings directly at the point of sale. Customers are more likely to buy insurance products when they are bundled with utilities – for example, auto insurance when purchasing a new car. Another trend could be microinsurance, where customers opt to insure sub-parts, resulting in sales uplift and upsell/cross-sell opportunities. It also allows insurers to embed offers in partner channels, such as house insurance for properties hosted via Airbnb, where premiums are commensurate with the number of days the property was rented out. GenAI can make this transition seamless, opening a channel for insurers and policyholders to communicate in real time with minimal hold-ups.
- Friction-Free Workflows: Post-pandemic, insurers put their weight behind digitizing processes to streamline customer onboarding, underwriting and claims processing. Despite tangible benefits, there is still scope to reduce latent friction. For instance, a customer fills in information such as name, age, address and bank details while signing up for a policy. Instead, by leveraging LLMs with access to third-party databases (enterprise or government), these details can be auto-populated with a customer’s Social Security number, reducing the number of required input fields. Similarly, the insurer can leverage GenAI to assess gaps in claims settlement, request additional documentation, analyze it in real time and significantly accelerate the time to closure.
The Long Haul
Before getting started with GenAI, insurers must factor in the following caveats:
- Consent-First Data Usage: Personally identifiable information (PII) and behavioral data are used to dynamically price in risk and offer customizations. It is also used to group customers into cohorts, influencing decisions on who gets left out of the insurance fold. It is critical to secure explicit consent to use customer data for decisions that may directly affect them, build guardrails to only use data that has been consented to and for the purpose it was collected and purge the data if consent is revoked.
- Regulatory Oversight: Weighing GenAI’s gains against potential misuse, regulators are pushing for ethical, transparent and responsible use of AI. Insurers must ensure the AI models or LLMs are trained on data free from bias – something that needs to be checked, remedied and reinforced with model validation. Insurers should also maintain audit trails of periodic model testing for compliance.
- Data Security: Because they collect hordes of PIIs, insurers have always been a prime target for cyber-criminals. A booming ecosystem of third-party intermediaries and non-industry players increases failure points exponentially. It is no longer sufficient to secure internal IT and data against breaches or misuse; Insurers have a fiduciary duty to ensure data is protected as it moves across the value chain.
- Higher Integration: GenAI requires real-time data from alternate sources that are outside insurers’ control. For GenAI to scale up, insurers must forge strategic ties with external stakeholders to enable data interoperability among systems hosted in different environments. Open source is a way forward but remains a reach for traditional insurers.
Preparing the Deck: A Comparative Analysis With Other Industries
As the insurance sector navigates its journey through GenAI scale-up, it can help to take lessons on unique challenges and opportunities from similar initiatives in other industries.
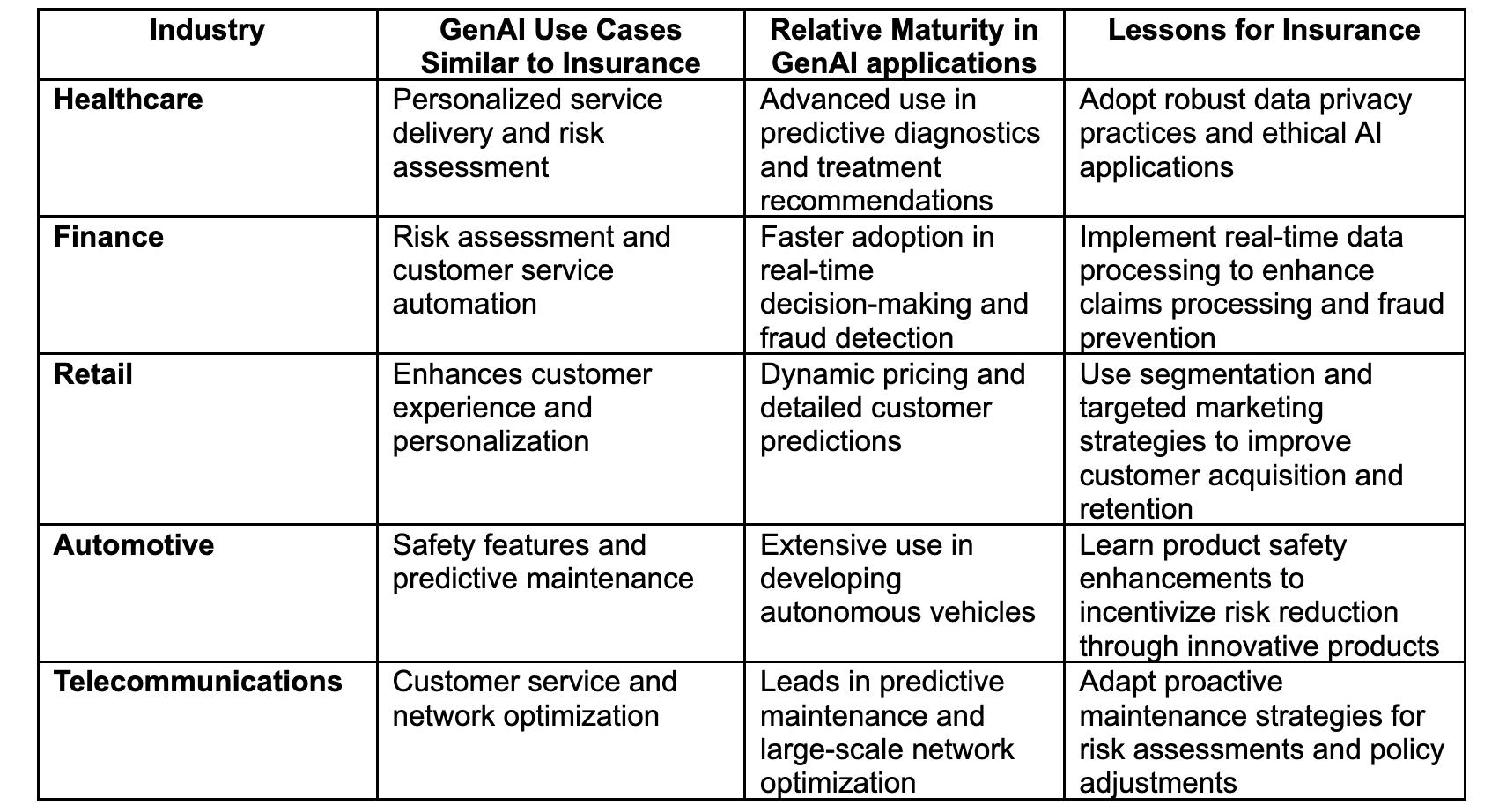
See also: The 10 Biggest Mistakes in AI Strategies
Business leaders may not say, "I did it because AI told me to do it.” That is why explainable, responsible and transparent AI is the first step and the hardest. Developing interpretable and explainable models calls for a well-documented data architecture that includes training data sources, decision-making criteria and logic and bias considerations.
The right technology partner can deliver a robust governance practice that addresses the ethical, social, privacy, regulatory and security aspects of AI adoption. They can also streamline consent-driven data usage with rule-based access policies, building secure and interoperable mechanisms for storing, using and transmitting data—enabling insurers to scale GenAI responsibly.