From historical claims data to hazard or policy data, insurance companies are sitting on an untapped goldmine of information, and only those that can convert this data into useful insights unleash its real value.
According to IBM, 47% of insurance businesses consider data and analytics a key to their competitive advantage. This percentage is projected to grow to 58% in a few years. This isn’t surprising, if we consider that data can help insurers enhance decision-making, contribute to innovation, and increase customer satisfaction and operational efficiency.
Let’s explore how to unlock the potential of big data with the help of analytics and machine learning technology.
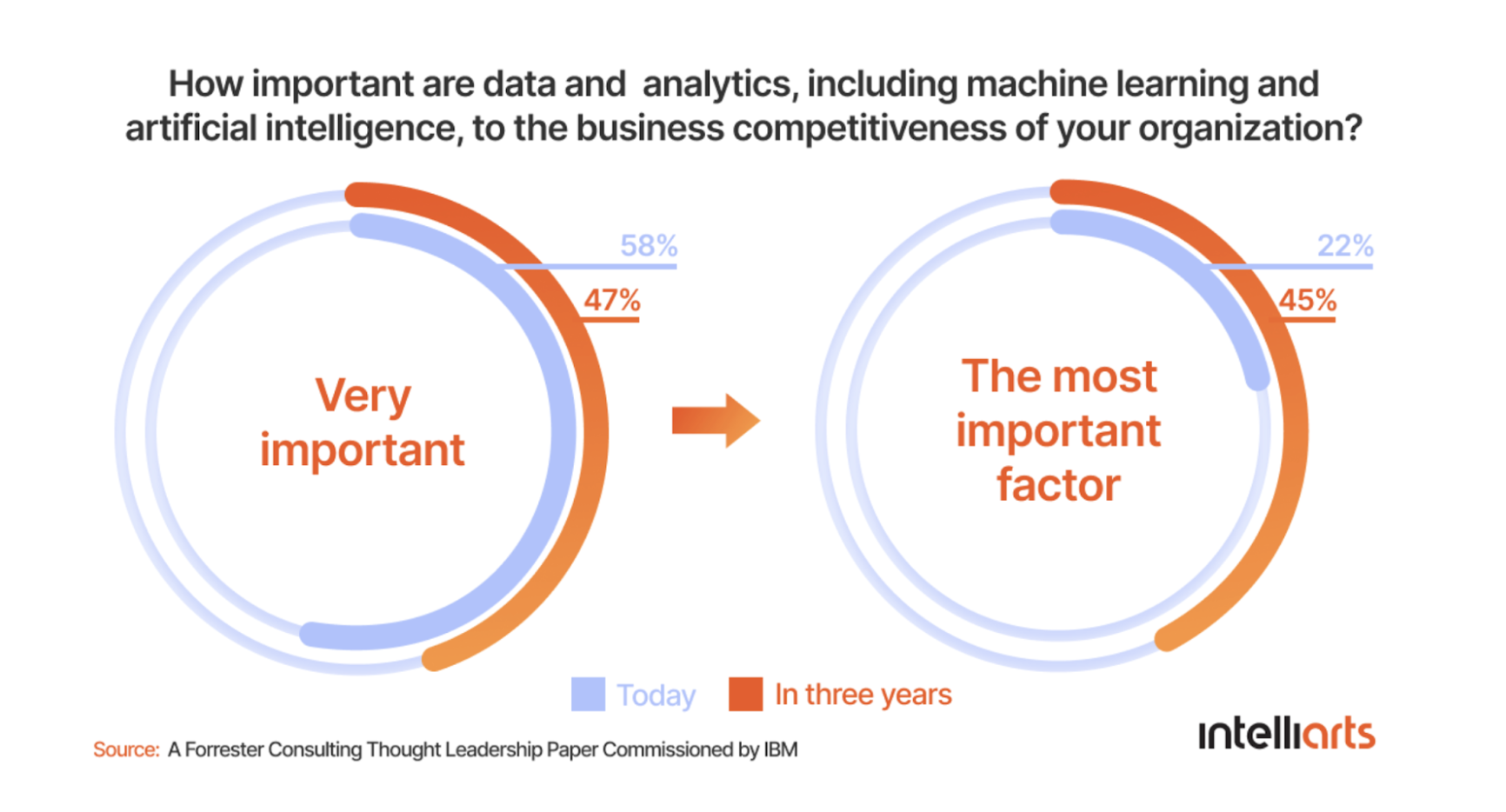
Five ways to get the advantage of insurance data
Research finds that from 10% to 55% of the tasks performed by insurers could and will be automated over the next decade, including claims, underwriting, marketing, and operations. We’ve already been witnessing a prominent shift in how insurers approach their business strategy, making an emphasis on data-driven insurance. The major values that data analytics and data science in insurance bring include the following:
Improved lead generation
Data and analytics, including unstructured data, offer valuable insights about customer behavior and market opportunities. Insurers can use this information to get a fuller picture of their ideal customer and their journey. As a result, an insurance company can personalize interactions with leads, provide in-context offers, and choose a marketing tactic depending on the value of the customer.
In particular, marketing insights received from insurance data could be used in:
- Building innovative products and services that would target specific customer segments.
- Choosing the best channels and touchpoints to contact the leads.
- Providing more personalized communication to attract leads, for example, by writing customized warm-up messages.
Here are some real-life examples:
- Paired with machine learning, data science has empowered a U.S.- based digital insurance agency to improve lead quality by 5% and insurance agent efficiency by 3%.
- French multinational insurer AXA relies on data analytics and predictive modeling to identify future product and service priorities for different customer segments. This big data strategy has allowed the company to increase its sales.
See also: 'Data as a Product' Strategy
Higher customer satisfaction and loyalty
No doubt, the secret weapon for business success is to keep customers happy. McKinsey says satisfied policyholders are 80% more likely to go for policy renewals. And there are lots of ways to increase customer satisfaction in insurance, from delivering services faster and more efficiently to making them more personalized.
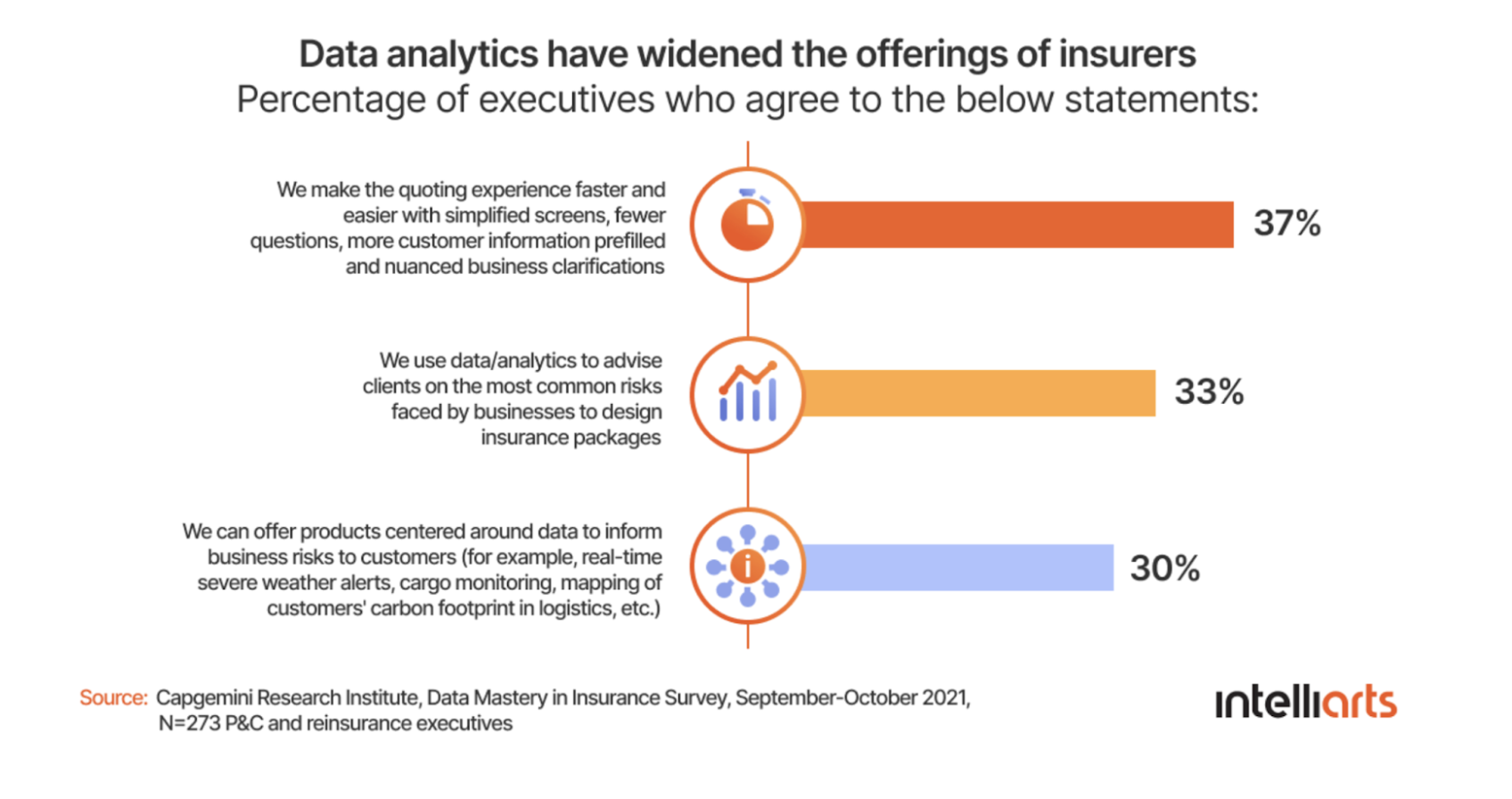
By looking through data trends and patterns, insurance companies can predict customer needs and discover opportunities to improve service. Data can also be used to predict customer churn. This way, an insurer could provide a timely, appropriate action to prevent customers from leaving.
Real-life examples:
- A U.S.-based insurance company, Prudential Finance, has started offering life insurance policies to HIV-positive customers after extensive data analysis found that they have a longer life span than previously known
- AIA's life insurance business in China uses data analytics to personalize insurance coverage for their customers. Out of thousands of possible coverage combinations, customers receive an insurance offer that is the closest to their needs
- A large U.S. insurer has analyzed available customer data, transaction data, and call-center interactions to develop new product offers. As a result, the company experienced a 40% increase in its retention rate.
New cross- and up-selling opportunities
Insurance data is also a substantial source of insights if the company seeks cross-selling and up-selling opportunities. With the aid of data science, an insurer can analyze customer behavior and track critical life events to target the customer with new types of policies and coverages that match the customer’s needs better.
The most illustrative example is the discount on bundled auto insurance when the company discovers that a policyholder’s child is near the driving age. Or the customer could receive an individual life insurance policy offer after the company discovers they have been exploring different options online. More advanced analytics, powered by machine learning, can even predict the likelihood of whether the customer accepts the offer.
Real-life examples:
- AEGON Hungary had access to tons of raw customer data, which the company planned to use for cross-selling. The insurer used statistical analysis and modeling solutions to connect customer life events to their insurance needs. As soon as it started to send personalized offerings to customers, the insurer improved its response rate by 78% and sales by 3%.
- John Hancock Financial, a life insurance company, has used an unusual data-driven approach to cross- and up-sell. The insurer offers a discount on new premiums based on how their customers are reducing their unhealthy and risky behaviors.
Claims optimization and fraud prevention
Combined with a machine learning model, data analytics allows insurers to handle claims faster and more accurately. Most importantly, it can help categorize risks and predict fraud likelihood in real time.
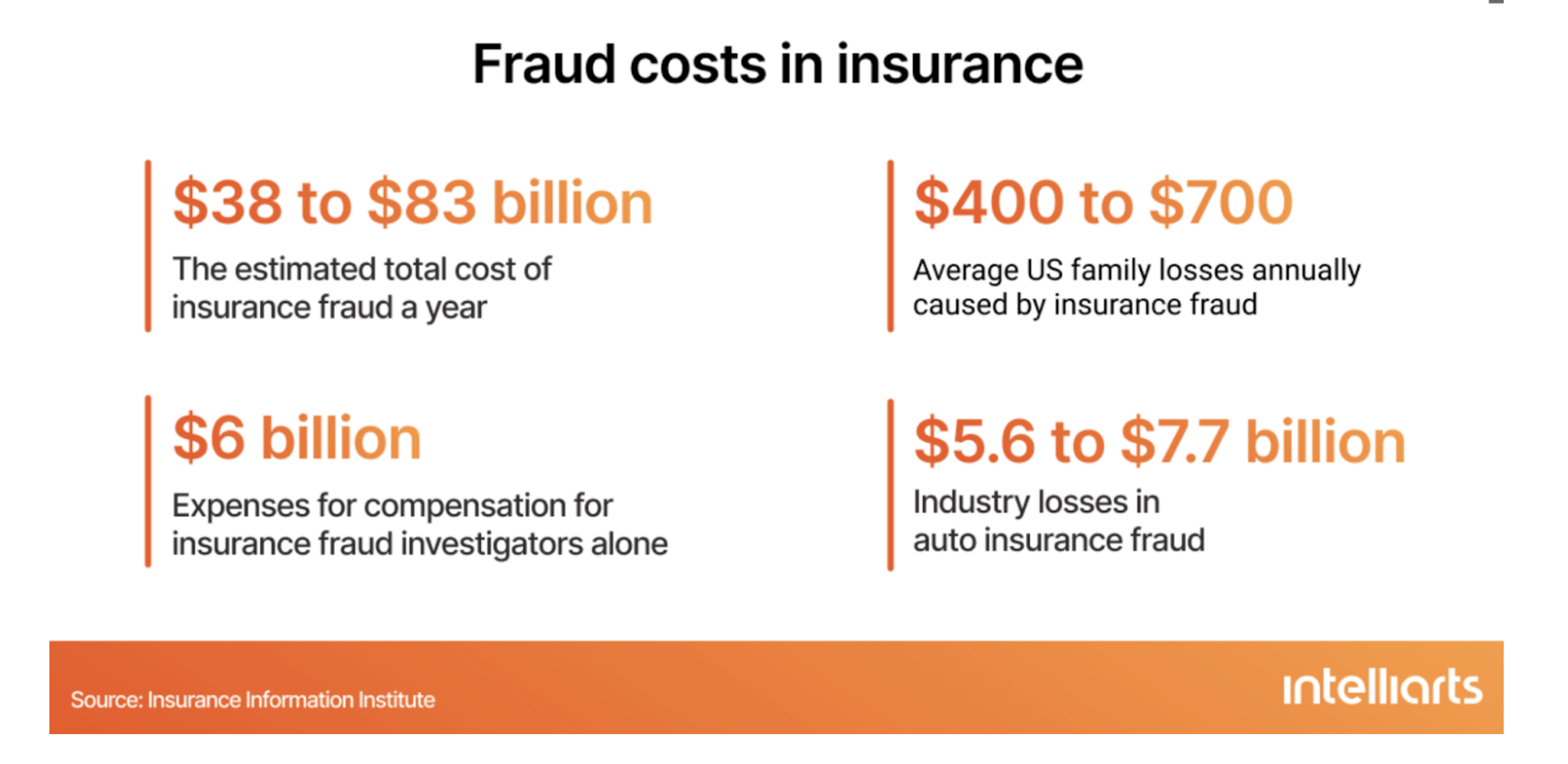
Built based on historical data, an ML-powered model can analyze data patterns to seek these trends in new claims. If any suspicious trend is noticed, the company will know immediately and inform investigators. The insurer can also use alternative data sources, such as geospatial data or social media. For example, geospatial data could be useful to check whether the policyholder is honest about accident details.
Real-life examples:
- After Santam Insurance deployed a predictive analytics solution for fraud detection, the company managed to save $2.5 million in payouts to fraudsters and almost $5 million in total repudiation.
- ZhongAn Technology, a Chinese insurance company, uses image recognition to mitigate claims fraud. Users are asked to upload a photo of their cracked phone screen, for example. ML-driven technology then helps the company decide whether the actual damage took place and allows automated claim processing.
- Allianz Insurance reports saving up to $4.5 million a year thanks to reducing fraud via data analytics.
- Poste Assicura, an Italian insurance company, estimates savings of 5% to 10% of claims since it introduced insurance data analytics to its fraud detection.
- Insurtech company Lemonade is using machine learning to compare claims against each other and detect fraud. More complex cases are transferred to an insurance investigator. The simpler ones are solved in a few seconds.
See also: Data-Driven Transformation
Improvements in insurance underwriting
Historically, underwriting was associated with a document-rich assessment process, which undermined any effort at automation. However, data and analytics have changed this approach, bringing automated data extraction, business intelligence, and predictive modeling to insurance underwriting.
In intelligent underwriting, insurers are able to prioritize submissions for quoting and start from the most valuable ones. In those sectors where the workload is the highest, such as in life insurance, carriers can decline the least profitable submissions at once and improve turnaround times.
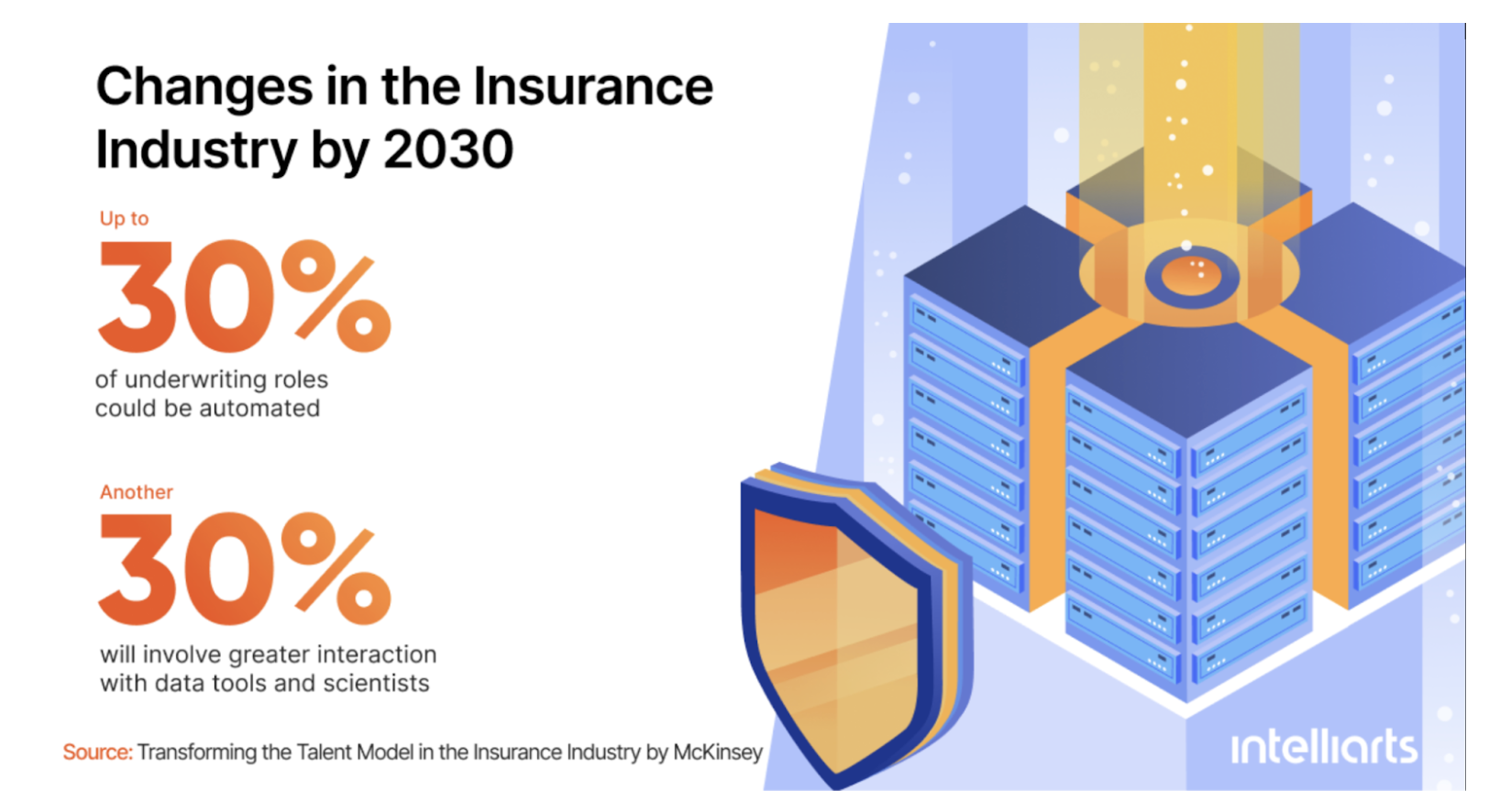
Insurance data can increase the accuracy of risk assessments. An insurance company would have access to more data sources to analyze the customer’s risk profile, such as credit agencies, social media, and third-party vendors. If, for example, it’s learned that the customer was involved in rough driving, they will receive a higher premium.
Real-life examples:
- A Scandinavian insurance company, Tryg A/S, used data analytics to check the effectiveness of its risk parameters. In 2021, the company discovered that 0.6% of its quotes needed reassessment because of risky behavior by those customers. Tryg A/S increased renewal prices for them.
- AIA Life Malaysia reduced its underwriting submission process to less than an hour by introducing a set of specific, profile-based questions on the digital platform.
- U.S.-based Allstate insurance company relies on telematics to collect and analyze mobility and driver data. The insurer then derives behavioral insights and optimizes premiums for auto insurance.
See also: 6 Steps for Cultivating a Data Culture
What should I do to unleash the power of my insurance data?
If you agree it’s the right time to use the data for the benefit of your organization, here are some recommendations on where to start:
- Invest in data analytics and big data: You won’t be able to get the advantage of your insurance data unless you put in order your data sources, data collection process, data preparation, and so on.
- Use machine learning: There are many use cases for ML in insurance, but the main idea is to build algorithms that will automatically process your insurance data, find patterns behind them, and help you improve decision-making.
- Build data expertise: At least half of insurance companies report the lack of data specialists in their organization, even though creating value from data is hardly possible without having one on your team. A good data scientist is worth their weight in gold; they don’t only have important technical skills but domain knowledge, too. A talented data scientist will see the broader picture of your business strategy and advise on how to solve your challenge with the help of data.
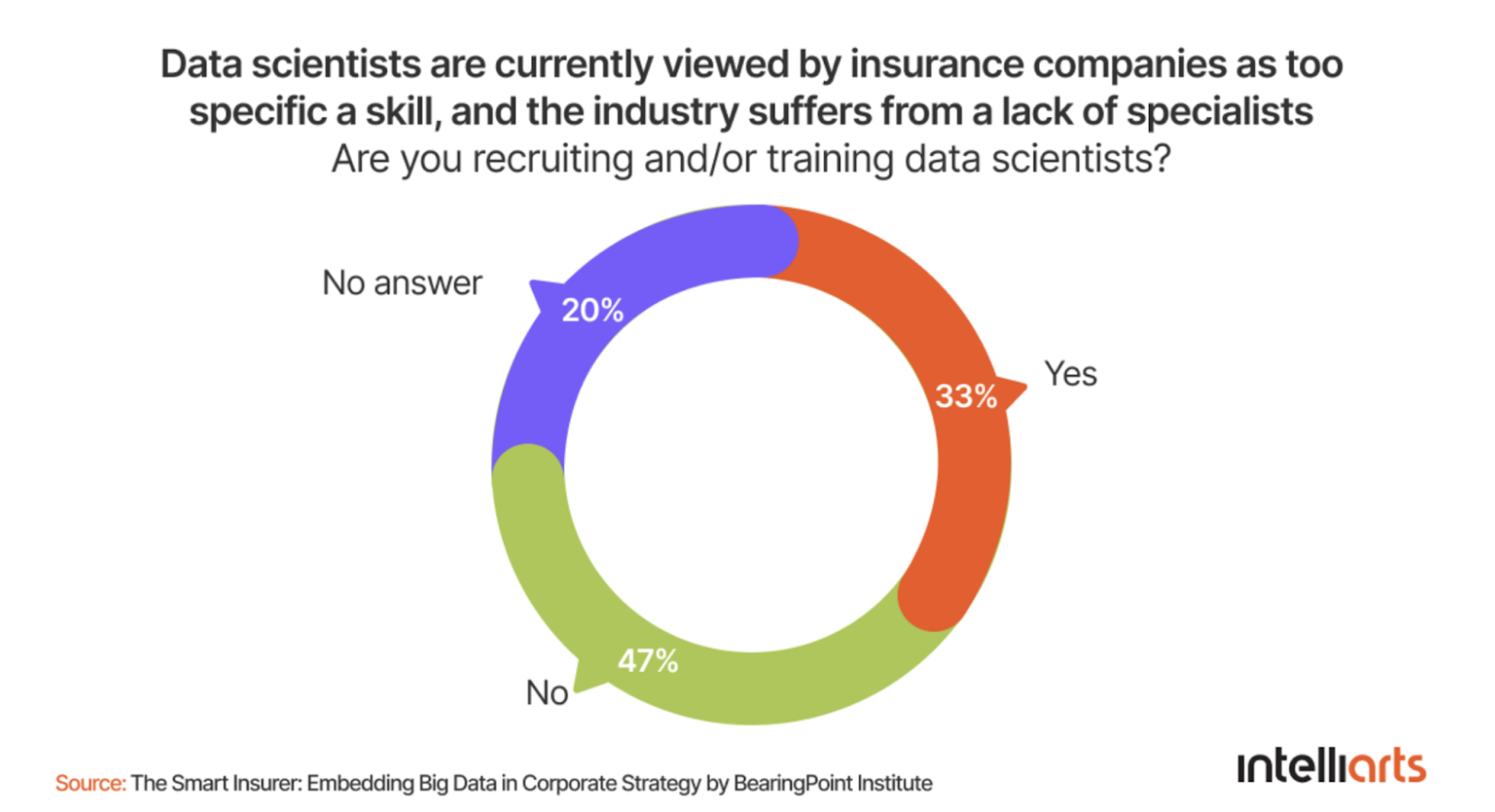
- Go paperless with OCR: Automatic data extraction is the first thing you should think of when improving your business processes in insurance. ML-based optical character recognition (OCR) reduces any manual entry and searches for sources and accelerates the insurer’s work.
- Pay attention to ethics and governance: Whenever data is involved, the issue of data governance, including data leakage and data privacy, becomes urgent. That’s why you should think about your company’s reputation and build customer trust and loyalty. Aside from committing to the principles of ethics and governance, try to walk the talk on ethics and be transparent with your customers when it comes to the use of data.
Wrap up
Having access to a large volume of insurance data is one thing. Being able to unlock the power of this data is something entirely different.
In the process of unlocking, the insurer can meet a range of challenges: from more global ones, such as how to introduce data analytics into your business strategy, to trivial ones, such as building data pipelines and machine learning models.
As a result of these efforts, the insurance businesses might get valuable insights: from powering up the company’s decision-making to handling claims most efficiently, optimizing premium rates, and much more.