Let’s consider the $300 billion U.S. auto insurance industry.
Driven by severe technician shortages, rising labor costs, repair complexities related to in-car technologies, and parts delays, the average cost of repair has more than doubled since 2019.
Independent body shops are declining in number as VC-backed multi-shop operators (MSOs) proliferate. MSOs are raising rates and limiting their participation in insurer direct-repair networks.
Capital formation in legal services and changing public sentiment on justice and fair settlement have increased the number of insurance claims going to litigation by 47% over the last three years.
Medical costs show no signs of slowing, and new Medicare Secondary Payer rules will shift more costs to auto insurers moving forward.
These developments feel more structural than cyclical. The same can be said for the homeowners and broader commercial markets, where climate perils loom and new species of cyber losses (e.g., CrowdStrike) evolve as fast as technology itself.
Insurance leaders are asking themselves the same questions they always have:
- Are our people working on the right things?
- Are our people working to the quality standard that’s needed?
- How fast are we cycling, operating, executing?
- How fast are we innovating? Moving with the market?
- What are our real costs related to all this?
What feels different these days is the urgency to find answers--concrete, quantifiable answers, in real time. Everyone has an opinion depending on their department, their patch. The goal is to generate a single source of operational truth based on how people are really working.
A new crop of software has emerged generating valuable operational intelligence without having to master query languages, statistical modeling, or the command line. Offerings from the likes of Skan, Mimica, Fortress IQ, Soroco, Automat, and Arkifi require minimal involvement from the IT department to install and create zero technology dependencies.
Installing this software on worker machines is way more philosophical and cultural than it is technical. The point to make is that it seems increasingly essential. As a baseball leadership team shunning player and positioning analytics operates at a disadvantage, so, too, an insurance leadership team.
There are three modes of implementation with this stuff. The first is load, don’t tell. Most employment agreements permit this. The second mode is load and tell--that is, let workers know it’s there, period. The third mode is load and share. Again, the baseball comparison is apt. Real metrics ranking team members published in real time can be a powerful motivator.
In the now, meaning the current quarter, the controllable input metrics generated by these tools are incredibly helpful driving efficiency and optimizing for, typically, cost. Here are examples in three key areas, working customer-back:
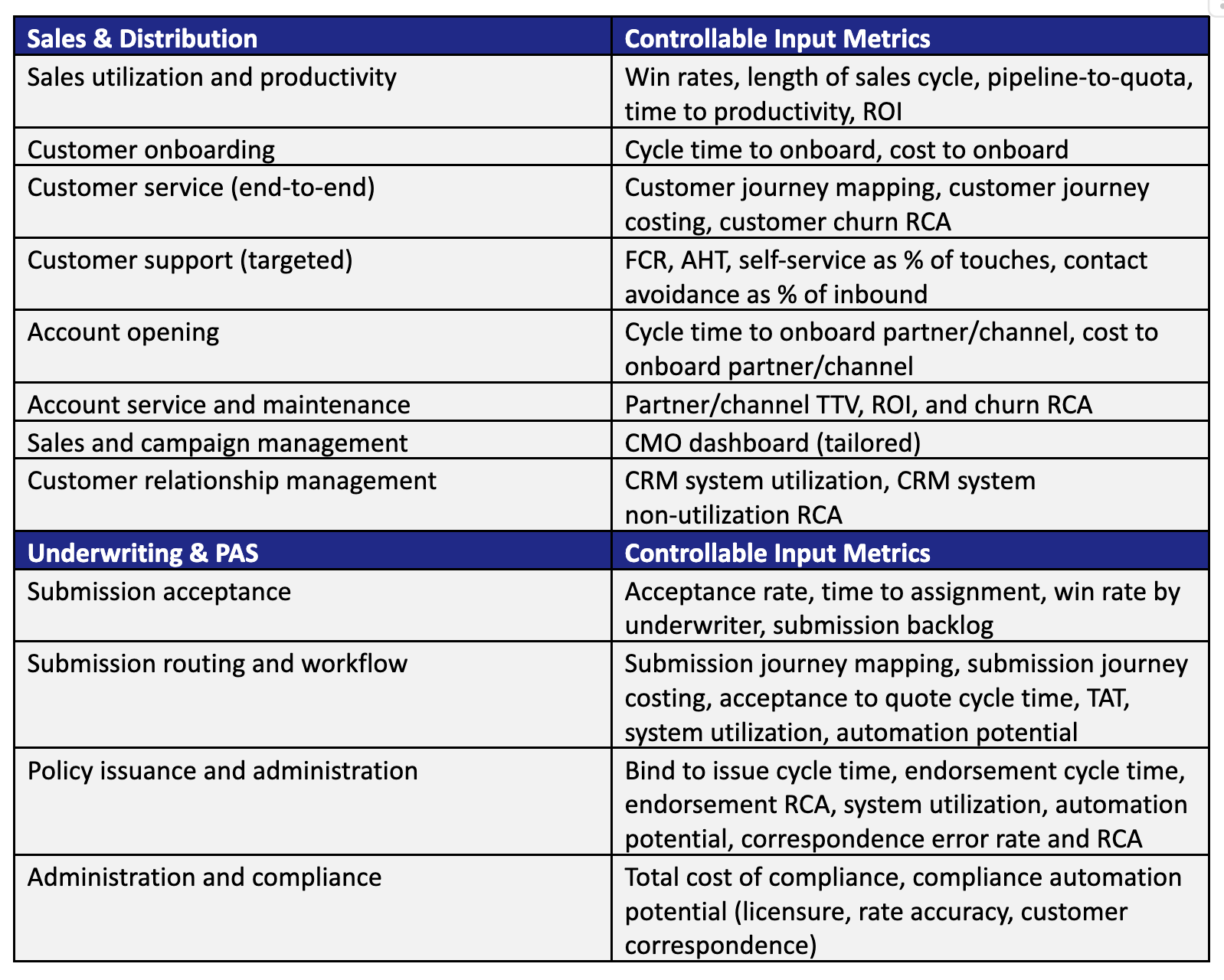
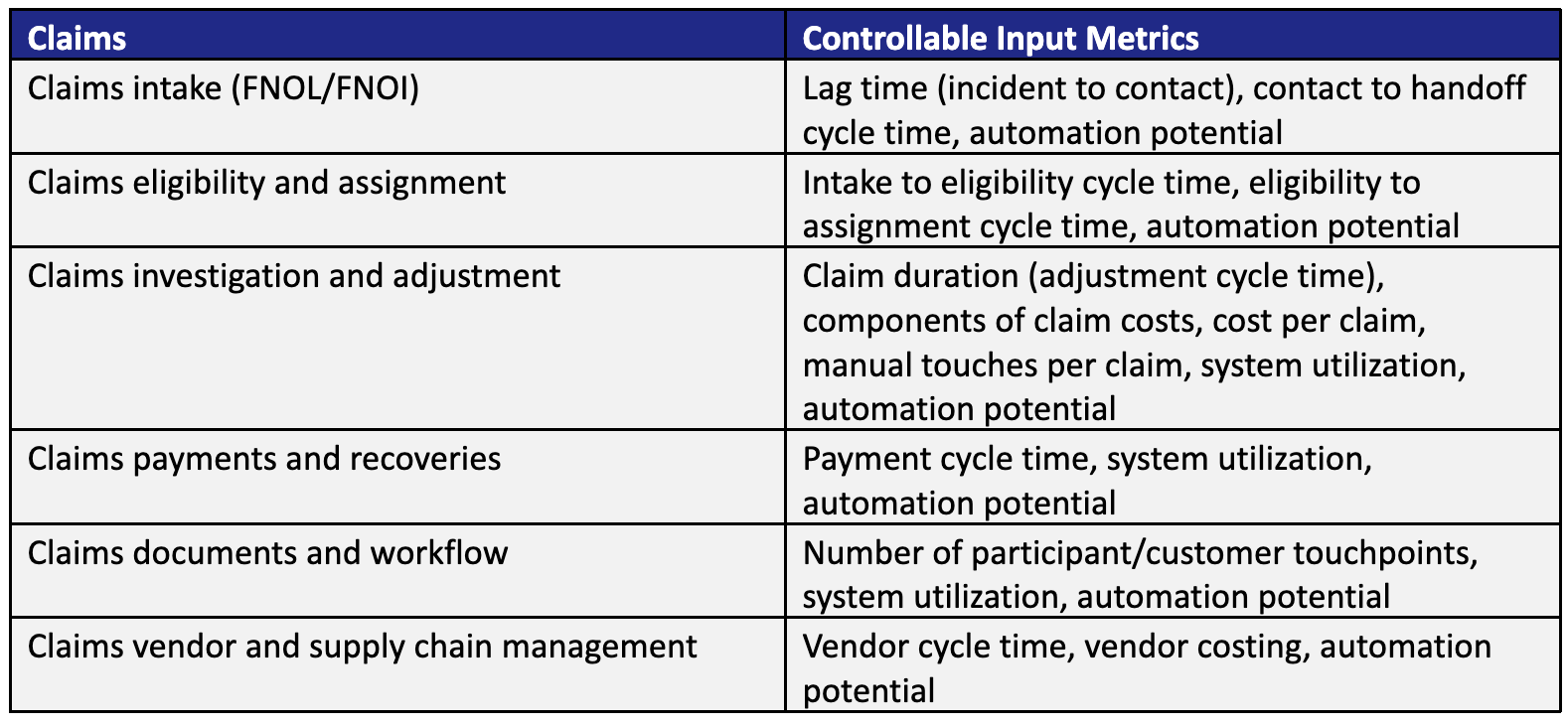
And then there’s enterprise AI. Most insurers are rightly reluctant to use open frontier models like ChatGPT and Anthropic’s Claude to do real work. Many carriers are moving in the direction of building custom models trained on their own data. Operational intelligence tools build a “digital twin” of the humans and systems they’re observing, useful for model training whenever it comes. Scaling laws state: the more data, the better the AI.
When the board asks, “What are we doing in AI?”, “building a digital twin of our operation for eventual in-house proprietary AI training” is never a bad answer. Especially when that same data drives operational gains paying for its creation.