“Despite the name artificial intelligence, there’s nothing artificial about it. As the industrial age amplified our arms and legs, the AI age is going to amplify our minds and our brains”. Manoj Saxena Executive Chairman of the Responsible AI Institute
This erudite quote encapsulates using AI to boost human insights and creativity. During the pandemic doctors placed expert decision making into AI datasets helping nurses deal with stress situationsi. Businesses turn to AI for first mover advantage, competitive edge, future of work, mundane business process automation, advanced analytics and human augmentation. Challenges abound around ethics, privacy, liability, cybersecurity, bias, transparency and loss of jobs. AI removes repetitive tasks so employees that learn will prosper as new jobs are created. Successful adoption centres around data integrity, verified trust and acting responsibly with exponential technology. Until recently AI had a relatively narrow focus looking at expert systems and performing singular tasks. Vendors competed for the best algorithmic approach with future promises of AI still in research labs and a perceived endgame in science fiction realms. Digitisation increased data volume, smart devices and systemic cyber risk bringing more AI technology out of the labs into production. Practitioners call this “third wave AI” and this paper looks at the implications of this paradigm shift on financial services moving from a pure algorithmic to a data driven approach as industries start to value data at the boardroom level. This innovation is recognised in global investment sectors where digitisation and AI lead the investment themes followed by renewable energy and cyber security both of which are becoming powered by AI. The stage is set. The following diagram show the AI development over a timeline.
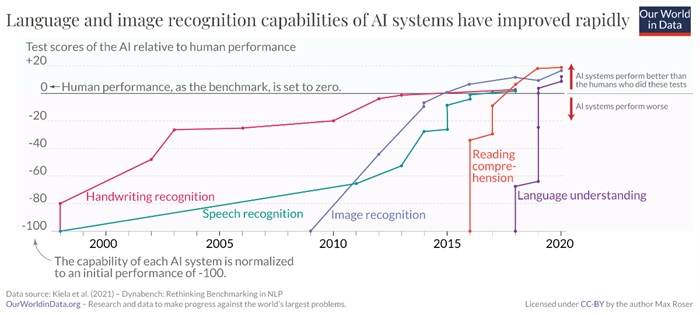
The first AI wave took an expert system approach by gathering information from subject domain specialists and ingesting experience-based rules. The second AI wave was driven by machine learning techniques to counteract risks of climate change, cybersecurity, digitization outcomes and better modelling results. Models were trained to predict outcomes but did not address uncertainty, being based on probability theory, Monte Carlo simulationsii, and essentially backward looking. The relevance of AI to spreadsheets became clear, as millions of users with modeling solutions extract data from spreadsheets to run random simulations stochastically to give probability and correlation of risk across enterprises. The result is a combination of first and second wave AI into workable, but not fully dependable, statistical models that in general lack forward looking and predictive capabilities. Moving to the third wave, machine learning datasets build transparent, underlying explanatory models with perception of uncertainty, using real-world scenarios with real time data. This co-pilot approach augments human creativity with machine learning and has implications for all industries. The insurance industry sits in the cross hairs for augmentation at the underwriting stage to better identify risk to appropriately price premiums and at the claims stage, reducing fraud and improving loss ratios by enabling more competitive pricing, resulting in faster parametric claim payments for customers. AI helps stock exchanges by tracking markets with clarity and understanding in real time, without delay or distortion, bringing boards and investors closer together. Banks get a better approach to credit analysis. All financial services benefit from customer experience, efficiency and are also challenged by the same risk register.
The articulation of “Causal AI” has the means to change models to utilise causality over correlation, explaining decisions by cause and effect. Causality has evaded AI progress to date and is the advancement of contextual third wave machine learning by unlocking black boxes and explaining decisions to accountable third parties such as regulators, expert witness courts, forensics, lawyers and auditors. Causal AI needs to be embedded in models and spreadsheets to get transparency. The insurance industry has recognized causality for time immemorial but represented it mathematically by correlation, a statistical method measuring dependency of two variables. Rational counterfactual explanations require machine learning and underlying causal models taking a contextual premise beyond statistical associations.
Until recently the great academic AI debate has been about Artificial General Intelligence (AGI), where machines start thinking like humans and how this intelligence leads to training machines to create new tasks versus Artificial Super Intelligence (ASI), a consensus of AI exceeding human cognition by total automation. The crux of the debate gets back to regulation, ethics and responsible AI so likely the AGI proposition will hold true as although AI self-awareness will emerge in machines, humans will remain in the loop as expected.
Emerging popularity of Generative AI (GenAI) third wave tools, like Open AI ChatGPTiii, portend to expand creativity and eventually enhance predictive modelling. AI is an umbrella term and detractors are saying that GenAI tools are not fully AI but act like a stochastic parrotiv as they are trained on volumes of data that are pre-generated and require continuous human input for mainstream adoption. The debates rage on, but announcements by Microsoft at WEF Davosv to build GenAI into apps and dashboards is a significant accelerator. If these tools are embedded in power-point or spreadsheet cells, then transparency and causality are ingrained in extant everyday work tools. This opens up a new dimension in the cloud computing and AI search engine race which has nudged Google to create equivalents. GenAI impact will help finance boards make informed investment decisions. By training these tools on financial data, companies leverage machine learning capabilities, identifying trends and patterns invisible to humans. It will take time before data sharing challenges allow all this data to be ingested as many regulations surround the use of data sharing and privacy, so AI needs an established code of conduct manifesto.
While ChatGPT may not replace the need for all human financial advice, at least in the near future, it may turbocharge service providers' ability to provide trusted advice and planning on the full range of financial services – ETBFSI.comvi
AI changes the landscape of cybersecurity by detection speed and predictive response to emerging threats, improving security. Conversely, GenAI can create malware scripts by bad actors for malicious purposes posing a risk to cybersecurity posture. Cyber risk needs to be mitigated at the design stage and cyber integrity maintained at all times as data used for training today will be the basis of machine learning in the future. This has to be done now, not added on, as discovery will be too late as AGI emerges and control of data could be lost.
Enterprise AI can be centralized (more risky), or decentralized with blockchain integration, revolving around data integrity (preferred). Transformation by machine learning is a boardroom issue. Complete enterprise AI solutions are emerging as low code no code, APIvii driven software to help companies leapfrog in AI whilst also addressing issues of reputational risk, solvency, brand awareness, operational resilience and data accuracy. AI regulation is a sensitive matter as algorithms shown to regulators reveal secret sauce IP. Technology itself is not regulated but instead the models, business processes and data flow that leads to customer outcomes, societal impact and bias assessment. The use of multi modal AI which holistically covers many media types (video, text and images), the reduction in social media deep-faking, embedding of AI software into hardware chips and use of synthetic data to train machines to avoid breaking privacy laws are enterprise developments that are evolving in the short term requiring corporate board governance, oversight and direction.
The 4th Industrial Revolutionviii is realised with Web3, Metaverse and AI. Blockchain protects the data layer and must underpin all data accessed by AI. Internet, cloud computing, mobility and blockchain have taken years to be adopted but the embedding of AI is just taking months. This explosion of data cum intelligence will shorten the time for quantum computing, causing the post quantumix standards (for encryption continuity) to accelerate. The mainstream adoption of the metaverse in parallel integration of AI into avatars and NFT’s impacts evolution of decentralized financex (DeFi) and the future of cryptocurrency. All these trends were alluded to at the WEF 2023. Ultimately it is about ensuring each piece of data has situational awareness. This is not a short-term issue about trusting third wave machine learning systems but a long-term issue as outputs are created on which future systems will be trained, so data standards must be mandatory otherwise we risk living in an ocean of false information sources and misleading data. Now is the time to act.
Financial services adopting third wave explainable AI will achieve transparency by evidence-based data decision-making and de-risking AI adoption fears with human input at each stage of the value chain to give confidence that systems are operating correctly. This drives transformation from a business outcome perspective with governance to stop automating bias at scale. Trust is maintained with full transparent explanation, cyber integrity, compliance to privacy/AI accountability laws thus mitigating residual risk from implementation and avoiding fines or reputational risk. AI implementation will require a holistic approach by companies led from the top, avoiding compliance silos. Like cyber, AI integrity needs to be by design, to get operational assurance using AI maturity modelsxi, NISTxii standards and certifications.
What of AI trends and the future? Sensors are a large part of the industrial world with consumer devices increasing to 80 billion by 2025xiii. The convergence of IOT and robotics change how humans interact in the real world. Autonomous transport, drones, smart agriculture equipment, surgical robots and 3-D-printed buildings will become pervasive, forcing open-source data sharing standards. Wearable device data can be ported directly to insurers, smart home and auto data made available through cloud computing so consumer device manufacturers can embed insurance at the OEM level. Public private partnerships will help create a common regulatory and cybersecurity framework around the AI paradigm.
“Artificial intelligence stirs our highest ambitions and deepest fears like few other technologies. It’s as if every gleaming and Promethean promise of machines able to perform tasks at speeds and with skills of which we can only dream carries with it a countervailing nightmare of human displacement and obsolescence. But despite recent A.I. breakthroughs in previously human-dominated realms of language and visual art — the prose compositions of the GPT-3 language model and visual creations of the DALL-E 2 system have drawn intense interest — our gravest concerns should probably be tempered”.
This article was originally published by International Insurance Society.
REFERENCES
[i] https://analycat.com/artificial-intelligence/uncertain-accountability-in-medicine/
[ii] https://en.wikipedia.org/wiki/Monte_Carlo_method
[iii] https://openai.com/
[iv] https://the-decoder.com/stochastic-parrot-or-world-model-how-large-language-models-learn/
[v] https://www.weforum.org/events/world-economic-forum-annual-meeting-2023
[vi] https://bfsi.economictimes.indiatimes.com/news/financial-services/how-chatgpt-the-new-ai-wonder-may-transform-bfsi/97083280
[vii] https://www.dataversity.net/what-are-ai-apis-and-how-do-they-work/
[viii] https://en.wikipedia.org/wiki/Fourth_Industrial_Revolution
[ix] https://en.wikipedia.org/wiki/Post-quantum_cryptography
[x] https://www.investopedia.com/decentralized-finance-defi-5113835
[xi] https://www.bmc.com/blogs/ai-maturity-models/
[xii] https://www.nist.gov/
[xiii] https://www.vebuso.com/2018/02/idc-80-billion-connected-devices-2025-generating-180-trillion-gb-data-iot-opportunities/
About the Author:
David Piesse is CRO of Cymar. David has held numerous positions in a 40-year career including Global Insurance Lead for SUN Microsystems, Asia Pacific Chairman for Unirisx, United Nations Risk Management Consultant, Canadian government roles and staring career in Lloyds of London and associated market. David is an Asia Pacific specialist having lived in Asia 30 years with educational background at the British Computer Society and the Chartered Insurance Institute.
Sponsored by ITL Partner: International Insurance Society