
Predictive Analytics for Self-Insureds
Predictive analytics is the tool that will help risk managers make better claim reduction decisions and produce actionable items with real cost savings now and in the future. |
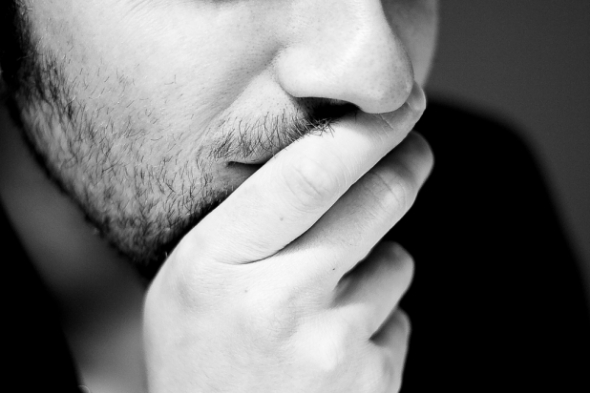

Predictive analytics is the tool that will help risk managers make better claim reduction decisions and produce actionable items with real cost savings now and in the future. |
Get Involved
Our authors are what set Insurance Thought Leadership apart.
|
Partner with us
We’d love to talk to you about how we can improve your marketing ROI.
|
Elizabeth Bart is an actuary in the Chicago office of Milliman. Prior to joining Milliman in 2007, Bart had four years of experience with a large international insurance company. Her area of expertise is property and casualty insurance, including loss reserving and ratemaking.