Dynamic pricing in insurance represents a paradigm shift from traditional pricing strategies to real-time, data-driven risk assessment and pricing optimization. By leveraging advanced analytics, artificial intelligence, Internet of Things sensors and telematics, insurers can now dynamically adjust premiums based on market conditions and behavioral patterns. This transformation enables personalized coverage and incentives for positive behavior and creates a virtuous cycle of risk reduction to deliver business value to policyholders and shareholders.
In 2023-24, as the insurance industry grappled with unprecedented climate-related catastrophes and geopolitical tensions, traditional actuarial pricing models faced challenges. When Hurricane Idalia struck Florida in August 2023, causing $3.6 billion in damages, insurers witnessed claim patterns that defied historical models. It was the strongest hurricane to hit Florida's Big Bend region in more than 125 years. Similarly, the Red Sea shipping crisis in early 2024 triggered a cascade of marine and cargo insurance disruptions, with premium rates surging. The "Change Healthcare" attack in February 2024 is an example of third-party ransomware exposure, where an insured company is affected by an attack on one of its service providers.
These events highlight limitations in traditional pricing strategy, which relies heavily on historical data, static risk factors, generalized linear models and experience rating. Also, changing risk exposures, customer behaviors, market conditions and increased competition necessitate the need for sophisticated pricing mechanisms to stay relevant and be perpetually adaptable to dynamic needs.
Current state and limitations
In the digital world, where risk postures and behaviors are changing dynamically and new risks are emerging, actuaries are facing twin objectives: to ensure profitability of the insurer and meet the obligations to insured through pricing strategies and technical provisions or reserving for payouts, respectively, in a competitive and regulated landscape. Today's pricing is anchored on generalized linear models (GLMs), historical claims data and catastrophic modeling. These traditional GLMs may not be able to handle very large or high-dimensional data sets and unstructured data (telematics, sensors, images, etc.), limiting their accuracy or effectiveness to capture behavioral changes and outliers, forecast claim cost trends and revise their assumptions that affect agility for rate revisions.
These methodologies operate on prolonged cycles such as annual rate reviews, quarterly loss development analysis and monthly exposure updates. The foundation of these relies on predetermined risk classifications, processed through batch systems with fixed rating engines and rigid schema requirements. With the emergence of dynamic risk exposures, as seen in Hurricane Idalia, geopolitical events and climate crisis, compounded by regulatory constraints requiring 60- to 90-day rate filing periods and growing demand for real-time pricing from digital distribution channels, the industry needs to evolve and adapt to new realities.
See also: How AI is Redefining Insurance Pricing Strategies
Dynamic pricing with reinforcement learning
Reinforcement learning (RL) transforms insurance pricing strategy from a static, retrospective process into a dynamic, forward-looking one. Unlike traditional methods, RL agents continuously learn from every pricing decision, interaction and claims outcome. It offers a solution by treating pricing as a sequential decision-making process where each pricing decision influences future customer behavior and market dynamics.
The RL approach to dynamic pricing involves the following components:
State Space (S) Engine:
- Demographics component to handle customer segmentation and risk profiling
- Claims analysis engine to process historical claims and identify patterns
- Market monitor to track the dynamics of competition and market conditions
- Portfolio tracker to monitor and maintain the book of business
Action Space (A) Controller:
- Price adjustment engine to manage premium modifications
- Discount manager to orchestrate incentives and promotion pricing
- Coverage modifier to handle policy/contract terms and conditions
Reward Function (R) Engine:
- Cost analyzer to monitor expenses such as acquisition costs and claims
- Revenue calculator to track income streams such as premium
- Performance metrics to measure business impact such as improvement in customer lifetime value, customer retention and profitability
It comprises a core engine, which includes:
- Environment simulator to generate hypotheses and synthetic scenarios to model customer behavior, claims and competitor responses to market conditions
- RL agent to implement the learning algorithm and employ dual learning approach, which includes active learning of customer behavior patterns, competitive response adaptation, etc., and offline learning via market scenario simulations
Data and Integration Layer:
- Real-time and batch data ingestion, processing and transforming data for RL models
- Feature store to maintain model inputs
- API gateway to manage external communications
- Service mesh component for microservices and interoperability
Decision Engine and Experience Layer:
- Policy network to determine optimal actions and pricing decisions
- Value network to evaluate state-action pairs and outcome prediction
- Experience layer to monitor and incorporate the feedback loop and improve the outcome
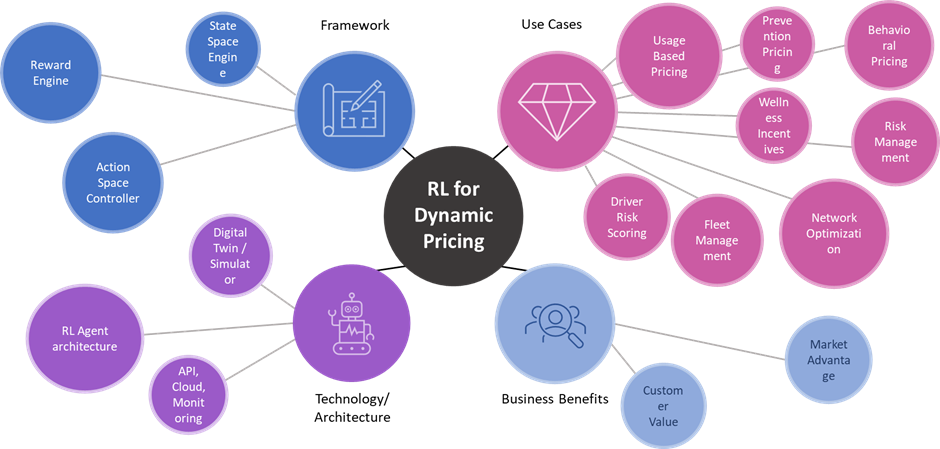
Figure 1: Dynamic Pricing Mind Map powered by RL
See also: How AI Could Set Premiums in Real Time
Value creation
Dynamic pricing empowers insurers to implement sophisticated premium adjustment frameworks that reflect dynamic risk profiles. In property and casualty insurance, telematics-based behavioral scoring models enable dynamic risk segmentation, incentives and improved loss ratios with engaged insureds. Similarly, wearable-based continuous monitoring creates opportunities for dynamic medical underwriting, risk prevention strategies and potentially reducing claims frequency and rewarding positive behavior change.
In commercial lines, integration of industrial sensor data enables dynamic exposure rating, as compared with static class rating. Also, analyzing insurance product/rate filings submitted by competitors to state regulators, using AI and large language models enables insurers to understand the proposed rate changes and objections raised by regulators. This granular approach to risk quantification not only optimizes technical pricing but also enables innovative product solutions driven by parametric triggers and usage-based insurance, leading to responsive risk management.
The Way Forward
As the industry evolves toward real-time risk assessment, dynamic pricing stands as a critical competitive differentiator. Insurers need to embrace an integrated ecosystem of data-driven pricing capabilities, which demands investment in robust data architecture, advanced analytics capabilities, seamless data exchange and personalization. The insurers that can effectively balance technical sophistication with market responsiveness, while maintaining pricing transparency and customer trust, will lead the frontier.